An artificial intelligence tool that can predict underlying neurobiological components of a person’s obesity risk by simply analyzing structural magnetic resonance imaging (sMRI). The findings highlight specific brain features (regions) linked to obesity, with potential towards developing brain-focused clinical interventions for individuals who are obese. Obesity increases the risk of brain diseases such as dementia and cognitive decline, which lead to neurobiological alterations. One key phenotype associated with obesity is body mass index (BMI). BMI is influenced by multiple causal pathways driven by behavioral, metabolic, and genetic factors. Traditional obesity prediction studies often rely on magnetic resonance imaging (MRI) voxel-based morphometry to correlate BMI with obesity-related clinical measurements and brain structure, predominantly grey matter volume (GMV). The research studies often show inconsistencies in regional GMV findings among obese patients, making it challenging to establish a clear relationship between obesity and brain structure. However, researchers point out that despite the predictive value of altered brain structure, identifying sub-cortical regions faces two challenges: the variability of identified brain regions across clinical studies and contradictory results between GMV and obesity within certain brain regions.
The Vision Lab team set out to tackle those challenges. They analyzed sMRI data from a major study, Human Connectome Project (HCP), that included patient information such as BMI, T1-weighted structural MRI, age, gender, race, ethnicity, level of education. Then they developed a model leveraging conformal prediction, to quantify uncertainty in the analysis to avoid contradiction and provide user-defined confidence, interpretability in the personalized prediction. The algorithm is unique because it can measure its own uncertainty and pinpoint specific brain areas, providing additional useful information for medical purposes. However, the researchers note that the results are still in the early stages. They plan to test the model further with neurocognitive assessments from different groups of people to ensure it works well in real-world medical settings.
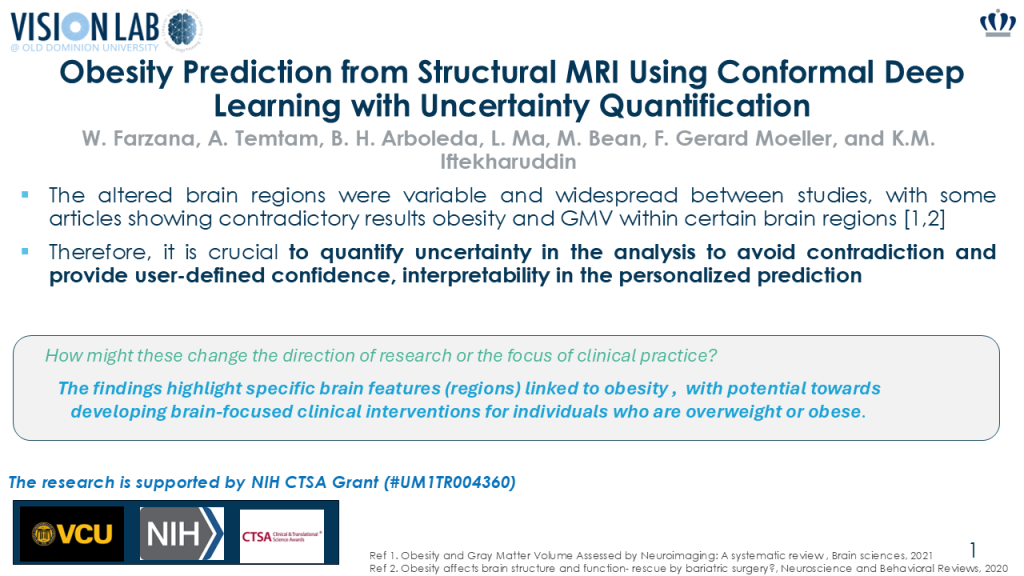
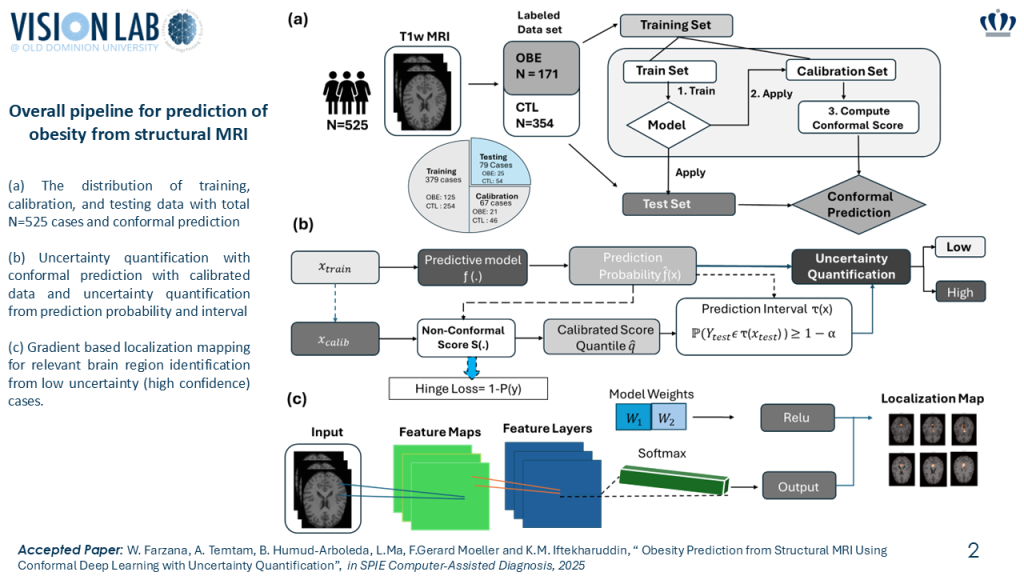
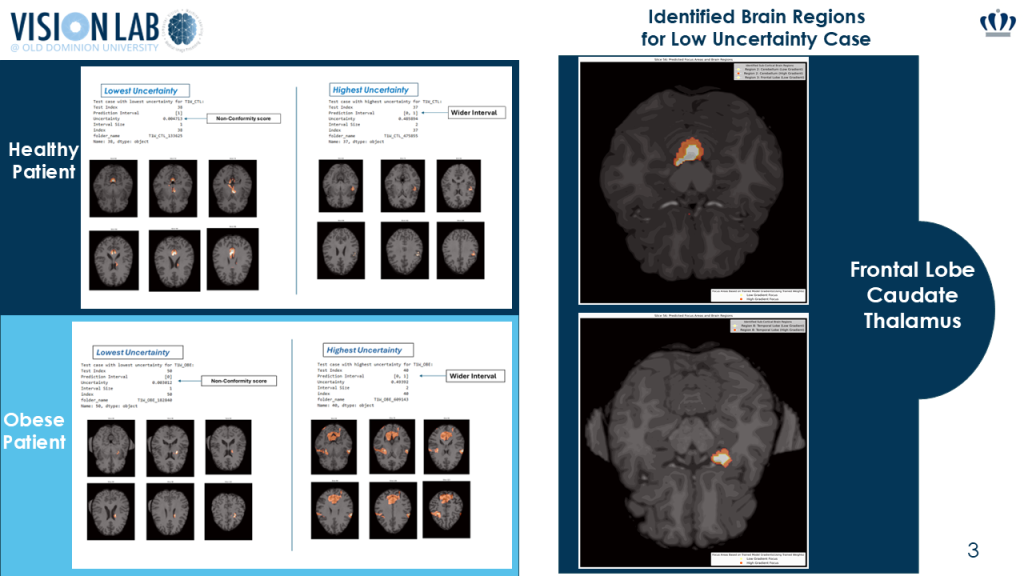